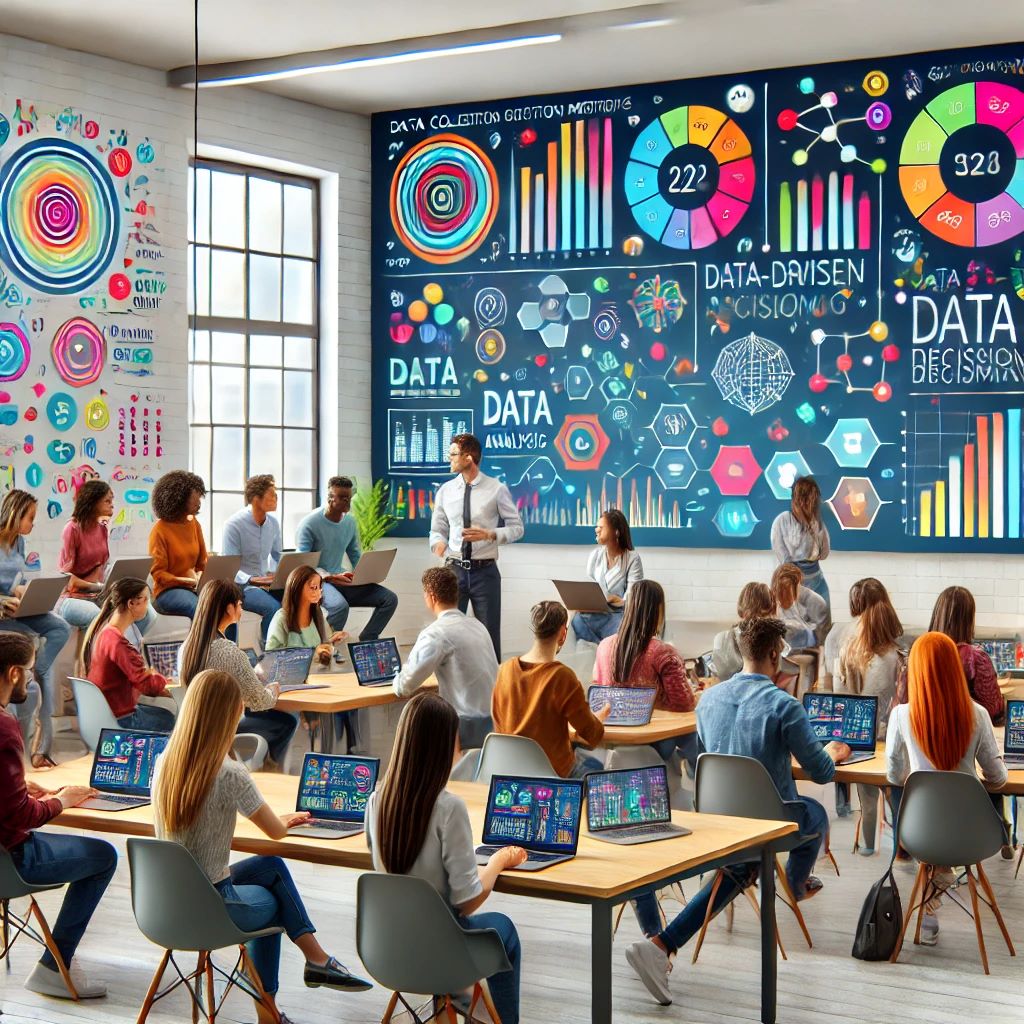
Week 9: Data-Driven Decision Making
Lesson Overview
This lesson will explore the critical role of data in strategic decision-making.
Students will learn about the importance of using data to drive decisions, various data collection and analysis methods, and how to integrate data analytics into the decision-making process effectively.
The goal is to equip students with the knowledge and skills to leverage data for making informed and strategic decisions in business.
Learning Objectives
By the end of this lesson, students will be able to:
- Understand the importance of data in strategic decision-making.
- Identify and apply various data collection methods relevant to business contexts.
- Analyze data using appropriate analytical tools and techniques.
- Integrate data-driven insights into the decision-making process.
- Evaluate real-world case studies to see how data-driven decision-making is applied in practice.
Key Concepts and Terminology
- Data-Driven Decision Making (DDDM): The process of making decisions based on data analysis and interpretation.
- Big Data: Large and complex data sets that require advanced tools for analysis.
- Data Collection Methods: Techniques used to gather data, such as surveys, interviews, observation, and digital analytics.
- Data Analysis: The process of inspecting, cleaning, and modeling data to discover useful information and support decision-making.
- Business Intelligence (BI): Technologies and practices for the collection, integration, analysis, and presentation of business information.
- Data Analytics: The science of analyzing raw data to make conclusions about that information.
Lesson Content
1. Introduction to Data-Driven Decision Making
Definition and Explanation:
- Data-Driven Decision Making (DDDM) refers to the process of making organizational decisions based on actual data rather than intuition or observation alone.
- The approach helps reduce biases, enhance objectivity, and improve the accuracy of business decisions.
Importance of Data in Strategic Decision Making:
- Data provides insights into market trends, customer behavior, and operational performance.
- Enables organizations to make informed decisions that are aligned with business objectives.
- Helps identify risks and opportunities, leading to more effective strategy formulation.
- Enhances transparency and accountability in decision-making processes.
Discussion:
- Engage students in a discussion on how data has transformed decision-making in various industries.
- Share examples of organizations that have successfully implemented data-driven decision-making strategies.
2. Data Collection Methods
Overview of Data Collection:
- Data collection is the process of gathering and measuring information on variables of interest in a systematic fashion.
- The quality of data collected directly impacts the accuracy of the decisions made.
Primary Data Collection Methods:
Surveys and Questionnaires:
- Used to gather information from a large audience quickly.
- Types: Online surveys, paper surveys, face-to-face interviews.
- Strengths: Cost-effective, scalable.
- Limitations: Response bias, low response rates.
Interviews:
- In-depth data collection method involving direct interaction.
- Types: Structured, semi-structured, unstructured interviews.
- Strengths: Rich, detailed information.
- Limitations: Time-consuming, potential interviewer bias.
Observations:
- Collecting data by observing subjects in their natural environment.
- Types: Participant observation, non-participant observation.
- Strengths: Real-time data, no recall bias.
- Limitations: Observer bias, time-intensive.
Focus Groups:
- Group discussions guided by a facilitator to gather qualitative data.
- Strengths: Interactive, deep insights.
- Limitations: Groupthink, potential for dominant voices to skew results.
Secondary Data Collection Methods:
Digital Analytics:
- Use of tools like Google Analytics to gather data on website traffic, user behavior, etc.
- Strengths: Large-scale, real-time data.
- Limitations: Requires expertise to interpret data accurately.
Public Data Sources:
- Government reports, industry publications, and academic research.
- Strengths: Often free or low-cost, reliable.
- Limitations: May not be specific to the organization’s needs.
Data Quality Considerations:
- Ensure data accuracy, completeness, reliability, and relevance.
- Discuss the importance of data validation and cleaning to maintain data integrity.
Activity:
- Students work in pairs to design a data collection plan for a hypothetical business scenario, such as launching a new product.
3. Data Analysis Methods
Overview of Data Analysis:
- Data analysis involves examining, cleaning, transforming, and modeling data to discover useful information, support decision-making, and answer research questions.
Quantitative Data Analysis:
Descriptive Statistics:
- Summarizes data to describe what has happened.
- Tools: Mean, median, mode, standard deviation, charts, and graphs.
- Application: Analyzing customer satisfaction scores.
Inferential Statistics:
- Makes inferences about a population based on a sample.
- Tools: Hypothesis testing, regression analysis, correlation analysis.
- Application: Predicting future sales based on historical data.
Predictive Analytics:
- Uses historical data to predict future outcomes.
- Tools: Machine learning algorithms, time series analysis, decision trees.
- Application: Forecasting demand for products.
Qualitative Data Analysis:
Thematic Analysis:
- Identifies patterns or themes within qualitative data.
- Application: Analyzing customer feedback to identify common concerns.
Content Analysis:
- Systematically categorizes and codes qualitative data.
- Application: Analyzing social media comments to understand brand perception.
Sentiment Analysis:
- Analyzes text data to determine the sentiment (positive, negative, neutral).
- Application: Assessing customer sentiment from online reviews.
Tools for Data Analysis:
- Excel: Widely used for basic data analysis and visualization.
- SPSS: Software for advanced statistical analysis.
- Tableau: Data visualization tool that helps create interactive and shareable dashboards.
- R/Python: Programming languages for advanced data analytics and machine learning.
Activity:
- Students apply a data analysis method to a sample dataset. They will summarize their findings using descriptive statistics and visualizations.
4. Integrating Data Analytics into Decision-Making Processes
Steps to Integrate Data Analytics:
Define the Business Problem:
- Clearly articulate the decision to be made or the problem to be solved.
Identify Data Requirements:
- Determine the type of data needed to inform the decision.
Collect and Analyze Data:
- Gather data using appropriate methods and analyze it using the chosen analytical tools.
Interpret and Present Insights:
- Translate data findings into actionable insights and present them in a clear and concise manner.
Make Data-Driven Decisions:
- Use the insights gained from data analysis to make informed decisions.
Monitor and Evaluate Outcomes:
- Track the impact of the decision and adjust strategies as needed based on new data.
Case Studies:
- Present real-world case studies where organizations successfully integrated data analytics into their decision-making processes.
- Example 1: A retail company using predictive analytics to optimize inventory management.
- Example 2: A healthcare organization using data to improve patient outcomes.
Group Activity:
- Students work in groups to develop a data-driven decision-making plan for a given business scenario. Each group will:
- Define the business problem.
- Identify data requirements.
- Outline a data collection and analysis plan.
- Present their plan and discuss how the data will inform the decision-making process.
5. Conclusion and Takeaways
Summary:
- Recap the importance of data in strategic decision-making.
- Highlight the various data collection and analysis methods discussed.
- Emphasize the steps involved in integrating data analytics into decision-making processes.
Reflection:
- Ask students to reflect on how they can apply data-driven decision-making in their own professional or academic projects.
Next Steps:
- Assign readings on advanced data analytics techniques and tools.
- Prepare for the next lesson, which will cover additional decision-making frameworks and their applications.
Additional Resources
Readings:
- “Competing on Analytics: The New Science of Winning” by Thomas H. Davenport and Jeanne G. Harris
- “Data Science for Business: What You Need to Know about Data Mining and Data-Analytic Thinking” by Foster Provost and Tom Fawcett
Tools:
- Excel and Google Sheets for basic data analysis
- SPSS and SAS for advanced statistical analysis
- Tableau and Power BI for data visualization
Online Resources:
- Coursera and edX courses on data analytics and business intelligence
- Harvard Business Review articles on data-driven decision making
Instructor Notes
- Preparation: Ensure availability of sample datasets and tools for data analysis activities.
- Engagement: Encourage active participation and real-world application of concepts.
- Follow-Up: Plan follow-up activities to reinforce the application of data-driven decision-making processes.
Visual Aids and Diagrams
- Data Collection Methods Chart: Display a chart summarizing different data collection methods.
- Data Analysis Flowchart: Provide a visual flowchart showing the steps involved in data analysis.
- Case Study Analysis Diagrams: Show how data was used in real-world case studies to inform decision-making.
Classroom Setup and Materials
- Smartboard or Projector: To display diagrams, charts, and example analyses.
- Group Workstations: Arrange seating to facilitate group discussions and activities.
- Handouts: Provide templates and worksheets for designing data collection plans and analyzing data.
- Laptops/Tablets: Ensure students have access to devices for data analysis